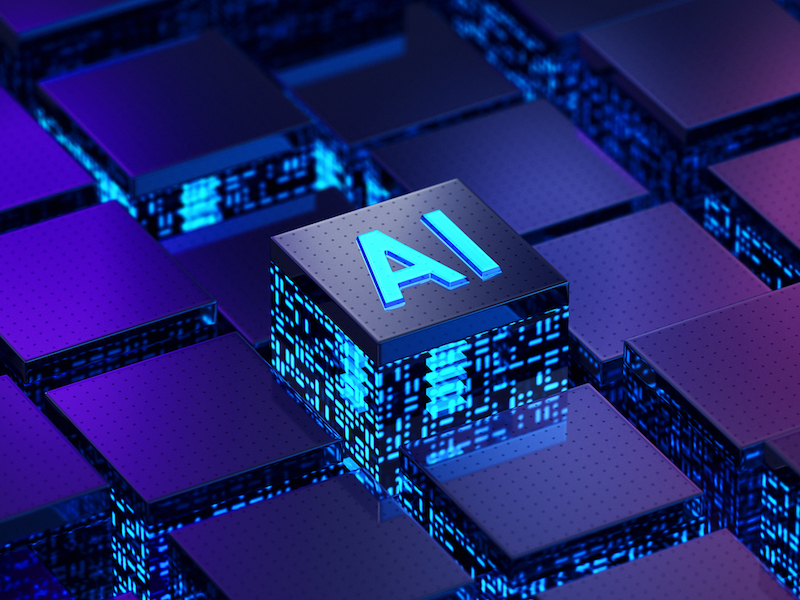
Asset managers began incorporating machine learning into their analysis and securities selection long before ChatGPT captured the collective imagination late last year. Several funds have employed artificial intelligence (AI) for years to analyze vast amounts of data, identify patterns and diversify portfolios.
Yet funds that hand over stock selection to the robots still are rare.
Gregory Allen, director of the Wadhwani Center for AI and Advanced Technologies with the Center for Strategic and International Studies in Washington, D.C., said AI is the latest tool for investment managers in their search for alpha.
“As an investor, where we’ve seen AI be extremely and obviously valuable is in extracting new value from existing data sets,” Allen said.
One of those data sets is quarterly conference calls, which have always existed but large language models like ChatGPT can now analyze at scale. And AI is capable of more than simply analyzing words in a transcript.
“They’ve determined there are statistical correlations between the ways that executives hesitate and dodge on answering questions,” Allen told an audience in November at the Portfolio Management Association of Canada’s annual conference in Toronto. And those hesitations are “actually correlated to market performance.”
AI is different from previous market-beating tools such as high-frequency trading, he said, in that “there are genuine strategic insights about the reality of the market that previously were perhaps only discoverable through human analysis.”
“That’s the sort of investor arms race we’re in,” Allen said.
Adrian de Valois-Franklin, CEO of Toronto-based Castle Ridge Asset Management Ltd. — a hedge fund that manages money for banks, endowments and sovereign wealth funds — said his firm’s proprietary AI technology, Wallace, allows managers to avoid the pitfalls of human bias and ego. “AI thinks differently from human managers, for better or for worse,” de Valois-Franklin said. “It can find behaviour and patterns in the market that are very different from the crowded trades everyone else is doing.”
De Valois-Franklin compared the process of examining the interrelationships of securities and identifying patterns with watching a flock of birds and noticing individual birds beginning to fly in a different direction.
“It’s seeing the individual securities that are showing independence of behaviour,” he said, and zeroing in on the information driving that behaviour, which may not be widely known to the market.
The advantage is the ability to develop strategies that are uncorrelated to what others are doing, he added.
In February 2020, Castle Ridge shifted from long positions to short positions on airlines and cruises, and went long on biotechnology and pharmaceuticals. “When the S&P [500] was down 35%, we were able to protect our clients and have meaningfully positive returns on the year within [our] market-neutral strategy,” de Valois-Franklin said.
While Castle Ridge doesn’t publicly share its performance, the firm was recognized as Best Emerging Manager at the 2021 HFM US Quant Awards.
Srikanth Iyer, managing director and head of i3 Investments with Guardian Capital LP, has been employing machine-learning models since 2018 to help predict a company’s dividend growth rate. His team’s algorithms aim to predict which stocks will have meaningful dividend growth — on an absolute basis and relative to the market — and which are more likely to cut dividends.
The $388-million Guardian i3 Global Dividend Growth Fund has posted a one-year return of 14.83% and a five-year return of 8.80%.
Iyer compared the machine-learning process to playing darts. But instead of the monkeys described in the long-running joke about stock picking, his analogy uses robots. Someone may throw 10 darts that land close to each other but nowhere near the bull’s-eye — an indication of bias. In another case, the darts may land closer to the target but spread around the board.
“Precision has bias; accuracy has variance. Both are sub-optimal,” Iyer said. “You don’t want a manager who’s precisely wrong, and you don’t want a manager who’s all over the place.”
A robot throwing thousands of darts every day, however, produces a volume of predictions that allows for averaging results with greater accuracy.
Some of the test cases for AI asset managers so far have been unconvincing. In 2017, Horizons ETFs Management (Canada) Inc. launched the Horizons Active AI Global Equity ETF (TSX: MIND), touted as the first AI-managed ETF. The fund closed in May 2022 with only $3.4 million in assets and a history of underperformance.
(Castle Ridge took over as sub-advisor of the MIND ETF in 2019, but the fund’s mandate was narrower than the one the firm applies in its own strategies.)
In the U.S., the US$103.7-million AI Powered Equity ETF (NYSE ARCA: AIEQ) from San Francisco-based EquBot LLC, which uses IBM Watson technology, has trailed its benchmark index and category since it launched in 2017. Its five-year total return is 4%, placing it in Morningstar Inc.’s bottom two quartiles each year since inception.
For now, Allen said he thinks AI will be confined to performing tasks and that it will be some time before investment managers trust automated trading systems without a human enabler.
De Valois-Franklin said there are various degrees of AI use in asset management. Some firms use AI as a screener, he said, with AI insights informing human decision-making. “There’s only a handful of players globally in the asset-management space that are doing pure-play, AI-type hedge funds,” he said.
Castle Ridge is one of those players. It uses what it calls “geno-synthetic algorithms” to analyze vast amounts of fundamental, technical, news and sentiment data.
“The big thing for us is the evolutionary side,” de Valois-Franklin said. “Most approaches in AI are still static.”
Deep learning and neural networks are great for teaching a computer how to recognize a face or play chess, he said. The problem comes when the rules change, as happens in financial markets, and the AI has to adapt.
Castle Ridge’s Wallace AI performs a “survival of the fittest” process for portfolio managers. It creates hundreds of virtual portfolio managers, each slightly different, and assesses whether a new manager is better or worse positioned for the current market environment, for which it uses a one-week horizon.
“If it’s better, it increases the probability that that manager breeds another, and it does this for tens of thousands of generations at a time,” de Valois-Franklin said.
Since ChatGPT went mainstream last year, many firms feel they need to adopt some kind of machine-learning strategy. De Valois-Franklin predicts a “disillusionment cycle,” in which firms try out AI strategies based on neural networks and abandon them when they aren’t successful.
A report this year from the CFA Institute noted the challenge of recruiting people with expertise in AI, machine learning and big data. The shortage of talent “inhibits firms from expanding their analytical capabilities and raises the premium that practitioners with both finance and data science experience can demand,” it stated.
The report added that AI applications are “still at a formative stage,” with few firms deploying the technology at scale across their businesses.
Asset managers using AI are convinced of the merits, though.
“AI will not replace human beings,” Iyer said. “But human beings who use AI will replace human beings who don’t use AI.”
This article appears in the December issue of Investment Executive. Subscribe to the print edition, read the digital edition or read the articles online.